By Jack Forehand (@practicalquant) —
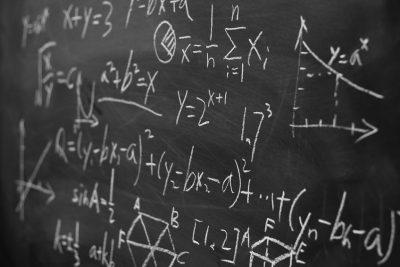
One of the major challenges that the finance community has faced over the years is how to explain what drives stock returns over time. If the market is efficient, then the only way to generate excess returns is to take more risk. But risk only makes sense to take if you get compensated for it. Determining which risks receive that compensation has been the subject of significant research in the academic community for many years.
When I was in college, the primary model that was taught was the Capital Asset Pricing model. CAPM is a very simple model that does a good job of getting across the point that there is a relationship between return and risk. It measures the risk of any stock using beta as its only risk factor. Beta is a function of both a stock’s volatility and its covariance with the market (how much they move in unison). According to this model, stocks with high betas would have an excess return over the market over time since they are riskier. As much as this model makes sense in theory, there is one major problem with it: it doesn’t work. In practice, stocks with high betas do not outperform the market.
In the early 90s, Eugene Fama and Ken French published their famous 3 factor model which added both value and size as risk factors. They found that small stocks and inexpensive stocks both generate excess return over time, but they do so because they are riskier. Their 3-factor model addressed many of the issues of CAPM and did a much better job of explaining long-term stock returns. Since then, they have improved their model further by adding investment and profitability as two additional factors.
Subsequent to the publication of the Fama and French papers, researchers have continued the search for the factors that best explain long-term stock returns to see if they could improve upon the Fama and French work. We are fortunate today to be joined by someone who has been a leader in that effort.
Dr. Lu Zhang is a professor of Finance and The John W. Galbreath Chair at Ohio State University. His research papers have challenged the status quo of traditional finance and have led to a better understanding of how assets are priced. He has also shown that many of the factors that investors rely on may not hold up as well as we think in the real world. In this interview, we find out why that is and discuss his research into what drives stock returns.
Jack: Thank you for taking the time to talk to us.
There has been significant debate about the risk factors that best explain long-term stock returns. It all started with CAPM and beta, but then Fama and French’s 3 factor model improved on that research by adding size and value as risk factors, and their 5-factor model further built upon that by adding profitability and investment. In your work, you eliminated the value and momentum factors and found that a model that just uses investment and profitability best explained returns. Can you talk about your Q factor model and the factors that it uses?
Lu: Sure, Jack. Thank you for your kind words. And thank you for reaching out.
The q-factor model is proposed in our 2015 paper published at Review of Financial Studies (Hou, Xue, and Zhang 2015). The q-factor model contains four factors, including the market, size, investment, and return on equity factors. I wish to point out the fact that the q-factor model predates the Fama-French (2015) 5-factor model, which is closely related to our q-factor model, by 3-6 years (see my 2016 article at Tsinghua Financial Review, Zhang 2016). In particular, although the factor construction differs somewhat, both models use the investment and profitability factors.
That said, I should acknowledge that the econometric design of the q-factor model and its empirical tests are heavily influenced by the Fama-French 3-factor model. Indeed, the objective of the q-factor model is to replace the 3-factor model as the next generation workhorse model for empirical asset pricing.
I am gratified to see that the myriad of new factor models subsequently proposed after our q-factor model:
- the Fama-French (2015, 2018) 5- and 6-factor models,
- the Stambaugh-Yuan (2017) “mispricing” factor model,
- the Daniel-Hirshleifer-Sun (2018) “behavioral” 3-factor model, and
- the Barillas-Shanken (2018) 6-factor model,
have all converged to the strong explanatory power of the investment and return on equity (Roe) factors. In particular, the Stambaugh-Yuan “mispricing” factors, once replicated via the traditional factor construction, have high correlations of 0.8 and 0.84 with our investment and Roe factors. And the Daniel-Hirshleifer-Sun factors, once replicated via the traditional approach, have also high correlations of 0.69 with our two q-factors. Stambaugh and Yuan as well as Daniel, Hirshleifer, and Sun have had to tap deep into the extreme distribution of stocks (with 20-60-20, instead of the common 30-40-30, breakpoints), by assigning more weights to microcaps, to show their “new” factors have “stronger” explanatory power than the q-factors. However, both conceptually and empirically, these factors are all very similar to our q-factors, as explained in our 2019 paper published at Review of Finance (Hou et al. 2019).
Given our deep respect and admiration for Fama, we view the Fama-French 6-factor model as our main competitor. The 6-factor model adds the momentum factor, UMD, into their 5-factor model. The following table updates the spanning regressions in Hou et al. (2019) between the q-factor model and the 6-factor model from January 1967 through December 2018:
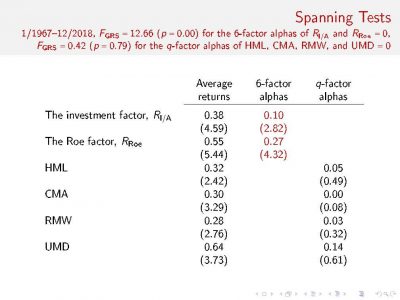
The table shows that the 6-factor model cannot subsume the q-factor premiums. The 6-factor alphas of our investment and Roe factors are 0.1% and 0.27% per month, respectively, both of which are significant at the 5% level. The Gibbons, Ross, and Shanken (1989) F-test on the null that the two alphas are jointly zero strongly rejects the 6-factor model. In contrast, the q-factor alphas of HML, CMA, RMW, and UMD are small, even tiny, up to 0.14%, with t-values all below one. The F-test on the null that the q-factor alphas of HML, CMA, RMW, and UMD are jointly zero cannot reject the q-factor model (p-value = 0.79).
Conceptually, most factor models are largely the results of pure data fitting, including the Fama-French 3-, 5-, and 6-factor models. In particular, the Fama-French (1993) 3-factor model is a largely empirical model aimed to fix the CAPM’s problems at the time. In an ultimate act of “Measurement without theory” (Koopmans 1947), the 6-factor model adds UMD into its lineup. Fama and French (2018) write: “We include momentum factors (somewhat reluctantly) now to satisfy insistent popular demand. We worry, however, that opening the game to factors that seem empirically robust but lack theoretical motivation has a destructive downside: the end of discipline that produces parsimonious models and the beginning of a dark age of data dredging that produces a long list of factors with little hope of sifting through them in a statistically reliable way (p. 237).” On the contrary, to me, the 6-factor paper is more like the end of the dark age that Fama and French started in 1993.
The q-factor model not only sets the new standard of empirical performance for factor models, more important, it silently questions the pure empiricism underlying the entire Fama-French research philosophy. In contrast to the 3-factor model, which started from the data, the q-factor model started from economic theory. I first realized back in 2005 that investment and profitability are fundamental driving forces in the cross section in the theoretical paper titled “Anomalies” (which I have never managed to publish). The investment and Roe factors just drop out of the math equations, naturally. I then set out the long journey of implementing this insight via the Fama-French factor approach, culminating in the q-factor paper published in 2015. The mighty struggle that I have gone through with the q-factor paper (described in my 2016 article) is a testimony of how hard it is to climb the steep learning curve of conducting high quality empirical work for someone like me who started out as a theorist. Along the other direction, Fama and French have never crossed the bridge to theory. Their theoretical effort of motivating the 5-factor model from the residual income valuation model is logically flawed, as explained in our 2019 paper.
I have recently laid out the theory of the investment CAPM in my 2017 article at European Financial Management (Zhang 2017) The theory says that firms with high Tobin’s q should invest more. As such, high investment firms tend to be growth firms, and low investment firms tend to be value firms. This intuition is why HML is redundant in the q-factor model, in the presence of the investment factor. In addition, earnings shocks are contemporaneously, positively correlated with shocks to stock prices. As such, price momentum winners tend to be earnings momentum winners that are more profitable, and price momentum losers tend to be earnings momentum losers that are less profitable. This intuition is why UMD is redundant in the q-factor model, in the presence of the Roe factor.
In summary, economically motivated, objectively validated, and parsimoniously designed, the q-factor model is an artful blend of theoretical clarity and empirical execution, well on the way to establishing itself as the next generation workhorse model. Our model forebodes the future of the best of asset pricing.
In contrast, the Fama-French 6-factor model is still playing the fossilized game of turning hard-to-explain anomalies into factors, as in the 3-factor model. While the 3-factor model represented a step forward toward scientific progress, the 6-factor model only serves to slow down, but shall never stop, history.
Jack: There continues to be significant debate in both the academic and practitioner community about whether the excess return of factors is purely risk-based or if part of the explanation is behavioral. I think most people would agree that the market is very efficient, which would mean that factors mostly outperform because they take more risk. But some also think there is an element of mispricing going on here. For example, some argue that part of the outperformance of value can be explained by the fact that investors tend to overreact to bad news, thus temporarily driving the prices of value stocks down below their intrinsic value. What are your thoughts on these behavioral arguments? Do you think a portion of the excess returns of factors can be explained by them?
Lu: I am open to the idea that a portion of anomaly returns can be due to mispricing. My team and I are about 3 months away from circulating a new working paper titled “The economics of security analysis.” We show in this paper that Warren Buffett’s Berkshire Hathaway earns 1.44% per month (t = 4.96) from February 1968 to December 2018. The q-factor model explains a big trunk of the average return, but still leaves an alpha of 0.64% (t = 2.45), and the q^5 alpha is even larger at 0.77% (t = 2.69). We interpret the evidence as a testimony of Buffett’s superior investing skills. As powerful as our factors are, our q-factor and q^5 models, which are based on simple, publicly available accounting information, cannot fully replicate Buffett’s astronomical success. More broadly, we interpret the evidence as consistent with the Grossman-Stiglitz (1980) theorem that a certain amount of arbitrage profits must exist in competitive equilibrium to compensate for arbitrageurs’ costly activities.
The heart of the EMH vs. behavioral finance debate is really quantitative. How much of the anomaly profits are due to rational forces, how much due to mispricing, and how much due to mismeasurement? When I was in graduate school (1998-2002), anomalies were typically interpreted as just mispricing. The most prominent examples are De Bondt and Thaler (1985), Ritter (1991), Jegadeesh and Titman (1993), Lakonishok, Shleifer, and Vishny (1994), and Sloan (1996). Fama and French (1993, 1996) argue that anomalies are compensation for risks in the context of the Intertemporal CAPM or the APT. However, since the sources of the risks have never been clearly specified, in theory or in practice, their argument has not been persuasive, not even for a die-hard EMH believer like myself.
I am by no means an impartial observer, but my sense is that the pendulum has swung back to the EMH side during the past 15 years. And my work might have played a role in this transformation.
The EMH vs. behavioral finance debate has traditionally been framed in the consumption CAPM framework, which is basically a more general formulation of the Sharpe-Lintner CAPM in the language of modern economic theory (Cochrane 2005). In this framework, the key determinant of expected returns is risks, defined in various ways. Since the consumption CAPM is typically rejected with the anomaly portfolios, empirical work has turned to the Fama-French 3-factor model as the workhorse model. But the interpretation of the common factors as risk- or mispricing-based is controversial, as seen in the covariances vs. characteristics debate between Daniel and Titman (1997) and Davis, Fama, and French (2000). The basic premise of this debate is that only risks matter in efficient markets. And to the extent that characteristics explain returns after controlling for risks, markets must be pricing risky assets wrong.
I defend EMH from a different perspective. In particular, I have ditched the concept of risks altogether, while retaining EMH. This is a bold step, as I am in effect saying that asset pricing theory in the past 50 years is incomplete. However, when you really think a bit, this step is quite natural. I am saying that the CAPM and the consumption CAPM approach the asset pricing problem only from the demand side, i.e., from the perspective of the buyers of risky securities. However, there is also the supply side, from the perspective of the suppliers of risky securities, without any information about the buyers. Asset pricing without the pricing kernel is the essence of the investment CAPM.
The investment CAPM is a mathematical restatement of Net Present Value (NPV) rule in corporate finance. The NPV rule is not controversial at all. The next logical step is to apply the NPV rule as an asset pricing theory. The economic reasoning for doing asset pricing from the supply-side NPV rule is exactly analogous to the reasoning for doing asset pricing as in the consumption CAPM from the demand-side permanent income theory of consumption. The big news is that in the investment CAPM from the supply side, it is firm characteristics that drive the expected returns. Risks are not unimportant, but their role is only in the background subsumed by characteristics.
EMH is in trouble only when paired with the consumption CAPM. When paired with the investment CAPM, EMH stands tall, as shown in the empirical performance of the q-factor model. The NPV rule is a standard neoclassical economic principle. I like simple economic models. When I can understand how the world works with a simple model, I hesitate to add ingredients like sentiment, mispricing, under-, and over-reaction. These ingredients are nebulous to conceptualize in theory and (close to) impossible to measure in practice. For example, they would imply that professional investors have been making systematic mistakes for 50 years, in the case of Ball and Brown’s (1968) post-earnings-announcement drift. To me, it is more natural to think of the earnings drift as an equilibrium phenomenon from the investment CAPM.
I agree with Fama (2017) when he says: “Asset pricing and market efficiency are forever joined at the hip (p. 6).” The consumption CAPM has many strong assumptions such as a representative investor, assumptions that make the model largely untestable. The investment CAPM has no such problems, and it seems to me it should be the next generation workhorse theoretical framework for asset pricing. More broadly, I agree with Fama (1970) on EMH. I just disagree with him on what theory and empirical models best represent EMH. But these are not fundamental disagreements. In particular, I feel that I might have done more than anyone else in my generation in defending EMH.
I do fundamentally disagree with Thaler and Shiller in that I think capital markets are working well, to the first order, as indicated in my large-scale empirical work. That said, I am keenly aware of several open gaps in the rational side of the academic literature. For example, we do not yet have a unified general equilibrium theory that can explain, quantitatively, the coexistence of value and momentum and/or the coexistence of investment and profitability premiums. For another example, a large fraction of profits for many anomaly strategies is realized on earnings announcement dates, giving rise to the impression of expectation errors. While the investment CAPM is qualitatively consistent with this pattern, careful empirical work needs to be done to evaluate the quantitative impact. If you ask me these questions again in five years, l may have better answers to these important questions.
Jack: In your paper Replicating Anomalies you looked at over 400 factors derived from published research and found that the vast majority of them do not hold up to statistical scrutiny when microcap companies are eliminated. Can you talk about the main conclusions that investors should take from this paper? What were the anomalies that held up best in your testing?
Lu: Dick Thaler sent the following tweet on May 8, 2017, shortly after we first circulated “Replicating anomalies” via NBER working paper series. In the tweet, Dick claims that we have excluded microcaps from our sample. Dick might have been suffering from the limited attention bias. We have in fact never excluded microcaps from our sample ever since the first draft of our work. We assign value-weights to microcaps in the first draft. We also furnish results from equal-weights in subsequent drafts.
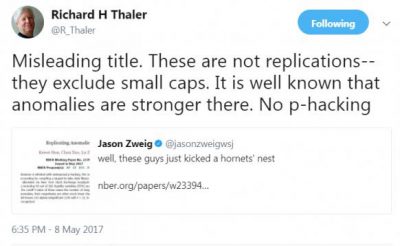
We report that out of 452 anomalies, the replication success rate (with |t| ≥ 1.96) is 35%, with microcaps in the sample. In the sample without microcaps, the replication rate drops to 30.5%. Across different categories of anomalies, momentum and investment anomalies replicate well, with 63.2% and 73.7% success rates. The value-versus-growth and profitability anomalies replicate fine, with 42% and 44.3% success rates, respectively.
The frictions anomalies replicate poorly, with only 3.8% success rate. Most of the liquidity variables are only priced within microcaps. Even with equal-weights, the replication rate for the frictions anomalies is less than 40%. There is a ton of fake news in this category. Note that we are not saying that liquidity, microstructure, and other frictions, such as transaction costs, do not matter in practice. What we are saying is that these friction variables are not nearly as important as value, momentum, investment, and profitability in terms of generating factor premiums in a broad universe of stocks.
The main lesson for investors is to always do replication before taking any results, published or unpublished, seriously. “Trust but verify.” Nowadays I just verify, if I am interested in any results that I come across. I have learned through pain to trust only results that survive independent, internal replication within my teams. The referee process in top academic journals leaves much to be desired. The self-correcting mechanism of science only works in the long run, and only for most important results.
Jack: One of the things I find most interesting is the intersection between the academic research and how it is applied in the real world of managing money. To help me better understand an academic paper when I read it, I like to try to envision what a portfolio managed using that research would look like in the real world. If you were to hypothetically manage an equity portfolio using the most significant conclusions from your research, what would it look like? What factors would it use to select stocks?
Lu: I would build mutual funds and/or ETF products based on the q-factors and the expected growth factor in our q^5 model, which augments the q-factor model with the expected growth factor (see our q^5 paper, Hou et al. 2018).
It is well known that DFA has built its line of investment products on the Fama-French size and value factors. We have learned much from the 3-factor model over the past 25 years. However, the 3-factor model is by now horribly obsolete, in terms of both economic foundation and empirical performance. I think I called it a “fossil” earlier in this interview. There seems to be a lot of room for the investment management industry to leverage on the latest developments in the academic world of finance.
The DFA investment philosophy makes sense to me. My understanding of it is to help investors better span the risk-return tradeoff in a multidimensional world. Now, the q-factor and the q^5 papers have never interpreted our factors as risk factors, only as common factors that capture large, common cross-sectional variations in stock returns. Unlike Fama and French (1993, 1996), the theorist in me hesitates to go for the risks interpretation, without either a clear understanding of economic mechanisms or evidence linking our factors to primitive risks facing the economy, such as business cycles or endogenous economic growth. However, for practice, the distinction between risk factors and common factors is only academic.
In this sense, the investment CAPM provides an equilibrium, economic foundation for active investment management in practice. It is “active” in the sense that it goes beyond the market portfolio, which only anchors the average return in the time series, even in theory. For the cross-sectional variation in the average return, the theory zeros in on investment, profitability, and the expected growth. The academic literature has recently debated on the merits of different profitability measures and has started on the merits of different investment measures. And our q^5 paper is among the first to even put the expected growth on the map. Graham and Dodd (1934) have long warned us about the danger of speculative growth. Yet, in theory the expected growth is an important part of the expected return. Lots of work remains in both academia and industry to better measure the key drivers of the expected returns.
Jack: The academic research on factor investing has come a long way in the past few decades and we continue to learn more about what drives long-term stock returns, Since you are leading the way in a lot of the new research that is coming out, I was wondering what topics you are looking into now and what you might be looking at going forward. What areas of research do you find the most interesting for the future?
Lu: I am actively working on a number of projects simultaneously, but three lines of work stand out as paramount. First, we are extending the q-factor model to the global data in a project on “Global q-factors.” The preliminary results indicate the investment and return on equity factors work in most countries. We are hoping to (finally!) finish a first draft by the end of this year. More broadly, I view global data as the future of empirical finance. Both behavioral finance and the investment CAPM have proposed economic explanations for anomalies, but it is hard to disentangle the two competing sets of explanations using the U.S. data alone. The cross-country data might provide a sufficient amount of variation in investor sophistication, limits to arbitrage, corporate governance, etc., for identification purposes.
Second, we are also pursuing a project on “The fundamental costs of capital.” It is well known that factor returns are noisy, and, as a result, the out-of-sample performance of the expected return estimates based factor models is weak (Fama and French 1997). In practice, one often just uses firm characteristics directly to forecast returns, but it is not clear how to form expected returns from reduced-form regressions.
An illustrious literature in accounting, pioneered by Gebhardt, Lee, and Swaminathan (2001), has built expected return estimates from accounting-based valuation models. However, it has become clear in the past two decades that these implied costs of capital do not forecast returns. We think the main reason is that these implied costs of capital are in fact internal rates of returns, which are constant over time by construction. Taken literally, they are not supposed to forecast returns, at least in the time series. We think the investment CAPM is a better theory for the 1-period-ahead expected return. The q-factor and q^5 models are basically linear factor approximations of this expected return. In the ongoing project on “The fundamental costs of capital,” we are experimenting to see if we can construct an expected return proxy directly from the nonlinear characteristics model via recursive structural estimation.
Finally, I am going back to my theoretical roots in a project on “An equilibrium theory of factors.” The goal of this project is to extend our disasters framework in a recent article (Bai et al. 2019) to explain both value and momentum as well as investment and profitability premiums in general equilibrium.
In short, a lot of work, and a lot of fun. Stay tuned.
Jack: Thank you again for taking the time to talk to us today. If investors want to find out more about you and your work, where are the best places to go?
Lu: My website is http://theinvestmentcapm.com/. You can also find me on Twitter at @zhanglu_osu.
Photo: Copyright: christianchan / 123RF Stock Photo
References
Bai, Hang, Kewei Hou, Howard Kung, Erica X. N. Li, and Lu Zhang, 2019, The CAPM strikes back? An equilibrium model with disasters, Journal of Financial Economics 131, 269-298.
Ball, Ray, and Philip Brown, 1968, An empirical evaluation of accounting income numbers, Journal of Accounting Research 6, 159-178.
Barillas, Francisco, and Jay Shanken, 2018, Comparing asset pricing models, Journal of Finance 73, 715-754.
Cochrane, John H., 2005, Asset Pricing, Revised Edition, Princeton University Press.
Daniel, Kent, David Hirshleifer, and Lin Sun, 2018, Short- and long-horizon behavioral factors, forthcoming, Review of Financial Studies.
Daniel, Kent, and Sheridan Titman, 1997, Evidence on the characteristics of cross sectional variation in stock returns, Journal of Finance 52, 1-33.
Davis, James L., Eugene F. Fama, and Kenneth R. French, 2000, Characteristics, covariances, and average returns: 1929 to 1997, Journal of Finance 55, 389-406.
De Bondt, Werner F. M., and Richard Thaler, 1985, Does the stock market overreact? Journal of Finance 40, 793-805.
Fama, Eugene F., 1970, Efficient capital markets: A review of theory and empirical work, Journal of Finance 25, 383-417.
Fama, Eugene F., 2017, My life in finance, in John H. Cochrane and Tobias J. Moskowitz (eds.), The Fama Portfolio: Selected Papers of Eugene F. Fama, The University of Chicago Press.
Fama, Eugene F., Kenneth R. French, 1993, Common risk factors in the returns on stocks and bonds, Journal of Financial Economics 33, 3-56.
Fama, Eugene F., Kenneth R. French, 1996, Multifactor explanations of asset pricing anomalies, Journal of Finance 51, 55-84.
Fama, Eugene F., Kenneth R. French, 1997, Industry costs of equity, Journal of Financial Economics 43, 153-193.
Fama, Eugene F., Kenneth R. French, 2015, A five-factor asset pricing model, Journal of Financial Economics 116, 1-22.
Fama, Eugene F., Kenneth R. French, 2018, Choosing factors, Journal of Financial Economics 128, 234-252.
Gebhardt, William R., Charles M. C. Lee, and Bhaskaran Swaminathan, 2001, Toward an implied cost of capital, Journal of Accounting Research 39, 135-176.
Gibbons, Michael R., Stephen A. Ross, and Jay Shanken, 1985, A test of the efficiency of a given portfolio, Econometrica 57, 1121-1152.
Graham, Benjamin, and David Dodd, 1934, Security Analysis, Whittlesey House.
Grossman, Sanford J., and Joseph E. Stiglitz, 1980, On the impossibility of informationally efficient markets, American Economic Review 70, 393-408.
Hou, Kewei, Haitao Mo, Chen Xue, and Lu Zhang, 2018, , working paper, The Ohio State University
Hou, Kewei, Haitao Mo, Chen Xue, and Lu Zhang, 2019, Which factors? Review of Finance 23, 1-35.
Hou, Kewei, Chen Xue, and Lu Zhang, 2015, Digesting anomalies: An investment approach, Review of Financial Studies 28, 650-705.
Hou, Kewei, Chen Xue, and Lu Zhang, 2019, Replicating anomalies, forthcoming, Review of Financial Studies.
Jegadeesh, Narasimhan, and Sheridan Titman, 1993, Returns to buying winners and selling losers: Implications for stock market efficiency, Journal of Finance 48, 65-91.
Koopmans, Tjalling C., 1947, Measurement without theory, Review of Economics and Statistics 29, 161-172.
Lakonishok, Josef, Andrei Shleifer, and Robert W. Vishny, 1994, Contrarian investment, extrapolation, and risk, Journal of Finance 49, 1541-1578.
Ritter, Jay R., 1991, The long-run performance of initial public offerings, Journal of Finance 46, 3-27.
Sloan, Richard G., 1996, Do stock prices fully reflect information in accruals and cash flows about future earnings, The Accounting Review 71, 289-315.
Stambaugh, Robert F., and Yu Yuan, 2017, Mispricing factors, Review of Financial Studies 30, 1270-1315.
Zhang, Lu, 2016, Factors war, Tsinghua Financial Review 37, 101-104.
Zhang, Lu, 2017, The investment CAPM, European Financial Management 23, 545-603.