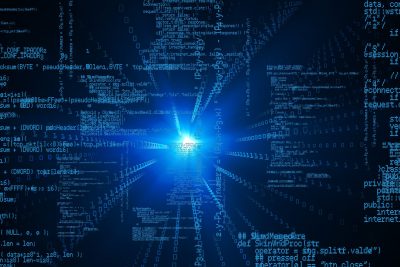
By Jack Forehand, CFA (@practicalquant)
Factor timing is a pretty controversial topic in the quantitative investing world. On one hand, we are all taught that we should buy low and sell high, so it seems intuitive to add exposure to factors that are out of favor. But in reality, it isn’t that simple and factor timing is much more difficult than it would seem in theory. This issue has also divided some of the smartest people in the factor investing community, with people like Rob Arnott of Research Affiliates being more supportive of a timing approach than others like Cliff Asness at
To try to get to the bottom of this, we are joined in this week’s interview by Nicolas Rabener of FactorResearch. Nicolas is one of my favorite follows on Twitter because his work is consistently rigorous and well thought out. In the interview, we discuss the various forms of factor timing and whether the strategy can generate excess returns for investors in the real world.
Jack: Thank you for taking the time to talk to us.
As I said in the intro, factor timing has been a controversial topic with some of the best minds in quantitative investing differing on whether it works. Before we get into the details of the individual methods of factor timing, I am wondering what your thoughts are on factor timing in general. Do you think it is possible to enhance the returns on a factor-based portfolio by timing factors?
Nicolas: Yes, and no. Factor timing is the equivalent of market timing, which is typically defined as forecasting returns based on discretionary or technical views. There is little evidence that such an approach works from any asset class, however, it also depends on the definition and framework employed.
For example, when we look at momentum strategies, regardless if modelled as time-series or cross-sectional, then we’re using historical prices to form conclusions about future prices. Similarly, value investors believe that cheap stocks will outperform expensive stocks. Both of these strategies are supported by volumes of academic research, but can also be viewed as forms of market timing, albeit by using systematic frameworks.
In general, we don’t believe that it is sensible to formulate views on future factor performance, but instead switch the perspective to factor riskiness. In contrast to forecasting returns, it is easier to predict risk, which is supported by academic literature. For example, volatility exhibits short-term autocorrelation and tends to cluster.
We therefore prefer to focus on factor risk management rather than factor timing. The goal of risk management is to improve the risk-adjusted returns, mainly by reducing the drawdowns, which can be severe as highlighted by the lost decade of the Value factor that we’re currently experiencing.
Jack: One of the more interesting possible ways to time factors is through macroeconomic data. For example, it seems intuitive to many that value stocks should do well when interest rates are higher and growth stocks should do better when they are lower since growth stocks are higher duration and more affected by a higher discount rate, but the evidence backing that up is questionable. In your series, Improving the Odds of Value, you took a look at some macro factors that could enhance the return of a value strategy over time. Do you think trying to time a factor like value using macro factors can enhance returns? Which macro factors have you found work best?
Nicolas: Our interest in macro-variables is primarily to gain insight into what is driving factor performance. Once we understand what is driving a factor, we can then try build a risk management framework, which focuses on determining when the environment for each of the different factors is more or less risky. As outlined previously, we are not trying to improve returns, but decrease drawdowns and volatility. We probably will have to give up some returns in the process, but investors will enjoy a smoother ride, which increases the likelihood of them sticking with the investment.
Let’s take the Value factor as a case study. If we rank the universe of stocks in the US for cheap stocks, regardless of the valuation metric, then we will end up with a basket of companies that face issues, which might be company- or industry-specific. Investing in these companies will be unpleasant as the news flow will be negative, they might be overleveraged, have negative sales and earnings growth, are rated “Sell” by brokers, etc. Most investors will want to hold exactly the opposite kind of stocks as it’s emotionally painful to hold cheap stocks. Investors that can bear the pain, should get rewarded for this service. However, they will only earn a premium if other investors will realize that these stocks are perhaps trading too cheaply and are happy to speculate on a turnaround of corporate fortunes. So the interesting question is: when do investors feel comfortable enough to take such a bet?
In the case of Value, it’s somewhat obvious: the factor will do better when the economy is growing, which would be a more supportive environment for troubled companies than if the economy is heading into a recession. Therefore we can observe that most Value factor performance is generated when real GDP and inflation are rising.
Unfortunately most of the macro-variables are practically useless as they are not available on a real time-basis, get revised frequently, and offer few data points for thorough analysis. Therefore it’s more interesting to explore variables that can be derived directly from the market such as skewness or the yield curve as these feature higher data quality.
We have yet to do further work, but our current research indicates that some market-derived technicals can be used to improve the risk-adjusted returns from factors by allocating tactically rather than strategically.
However, I should note that the challenge is not necessarily to derive a robust system that we can verify out-of-sample, but to actually follow the model. Our analysis suggests multi-year periods of zero allocations to certain factors. It’s questionable how many investors can implement and successfully execute such a framework.
Jack: Another method often used to time factors is their relative valuation. For example, if the spread between value and growth stocks is wide, as it is now, many believe that investors should add exposure to value since it is cheap on a relative basis. Some investors do the same thing with other factors like momentum and low volatility. What are your thoughts on timing factors via valuation?
Nicolas: We’ve done less work on relative and more on the absolute valuation, which can be measured by the difference in valuation multiples between the long and short portfolios of a factor. However, neither approach is particular useful for measuring the risk of factors on a stand-alone basis. Factors, like stocks, can be expensive for a long time.
However, an important technique in quantitative finance is to combine metrics as it reduces the model risk from being overly reliant on a single variable. Therefore we can use the absolute valuation in a multi-metric risk management framework for factor allocations. Despite being ineffective on a stand-alone basis, it does add value when combined with other metrics.
Jack: The final method commonly used to time factors is momentum. AQR, whose research has largely not supported factor timing, came out with a paper this year called Factor Momentum Everywhere, where they found that momentum could be used to time factors. In the summary of the paper, they said the following:
Factor momentum adds significant incremental performance to investment strategies that employ traditional momentum, industry momentum, value, and other commonly studied factors. Their results demonstrate that the momentum phenomenon is driven in large part by persistence in common return factors and not solely by persistence in idiosyncratic stock performance.
What are your views of using momentum to time factors? Do you think there is excess return to be gained by doing it that exceeds the transaction costs?
Nicolas: We published two research notes on factor momentum, which both showed positive excess returns. The first analysis described a long-short portfolio of factors selected by their 12-month performance and included transaction costs. The second analysis measured the time-series momentum of factors by using a short one-month lookback. However, although the excess returns were positive in both cases, the performance was not particular consistent across time and therefore attractive for investors.
However, similar to using valuation spreads, factor momentum is accretive as a component in a multi-metric model for measuring the riskiness of factors.
Jack: One of the big concerns many investors have about factors is crowding. As more and more investors adopt a factor-based approach, the concern is that they will become crowded and won’t work as well in the future as they have historically. You have developed an interesting factor crowding model that looks for factors that exhibit the characteristics that indicate potential crowding and looks at how that impacts the factors going forward. Can you talk about your research on factor crowding and the implications of it for investors? Do you think investors would be wise to avoid factors when they become crowded?
Nicolas: Crowding, regardless if for factors or asset classes in general, is a complex topic. A common misconception is that crowding is negative, but investors require others to also show interest and allocate capital to investments, as otherwise there will be no performance. An uncrowded asset is like a value-trap, not particular attractive.
Naturally there is the risk that at some point the entire market is long a certain asset and no buyers are left. If the sentiment shifts, then drawdowns are likely. However, frequently assets recover after a swift sell-off and continue to generate positive returns. We therefore define a crowded factor as having a higher probability of experiencing a drawdown, even if it has little implication on expected returns.
We developed a factor crowding model that measures five different metrics on a real-time basis and can indicate when factors are crowded, uncrowded, or in a neutral state. It depends on the model specifications, but factors are crowded less than 5% of the time.
Some investors have strategic factor allocations where this is useful for managing internal or client expectations while others trade factors tactically and have the flexibility to act on it. Either way, factor crowding should be measured as it forms an important component of a sound risk management framework.
Jack: Thank you again for taking the time to talk to us today. If investors want to follow your work, where are the best places for them to go?
Nicolas: Investors can peruse and subscribe to our research on our website, follow us on Twitter, or connect with me on LinkedIn. Thanks for having me!
Photo: Copyright: 123rf.com / wavebreakmediamicro
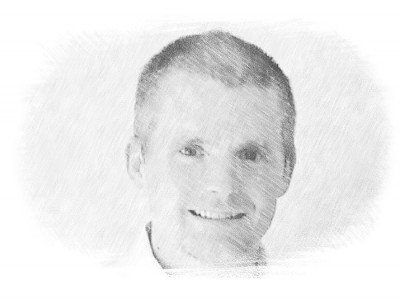