By Jack Forehand (@practicalquant)
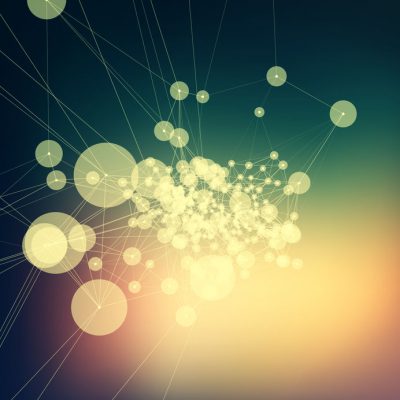
One of the most important things any investor can do is to challenge their own beliefs. Confirmation bias leads all of us to seek out opinions that agree with us and then use them to confirm what we already think to be true. That can be very dangerous, though, because you can end up missing obvious truths that are right in front of your eyes.
Those who read my articles will know that I am a big believer in factor investing. I often talk about why I think factor-based strategies will work over time and how the factors like value that have worked in the past are likely to continue to work in the future. In supporting that thesis, I will often cite people like Wes Gray and Jim O’Shaughnessy (both of which we have or will be inteviewing for this series), who present strong evidence to confirm what I already believe.
But there is another side to the argument.
What if the world has changed? What if the things that worked in the past won’t work as well in the future? What if the way we are all told to interpret facts about investing ends up being what matters more than the facts themselves?
To look at the other side of the coin, I am privileged to be able to interview Ben Hunt. Ben, along with his partner Rusty Guinn, is the founder of the Epsilon Theory blog. He also is the number one source I use to combat my own confirmation bias. No one is able to more eloquently explain why the world has changed, and why things may in fact be different this time than Ben. His work is extremely thought provoking and will likely cause you to also rethink some of your own beliefs about investing.
Just a note before we begin. This interview was transcribed from a phone conversation so please forgive any grammatical errors. Since I asked some follow up questions during the interview, there are also more than our standard five questions.
Jack: Thank you for taking the time to talk to us. And thank you for the great work you do at Epsilon Theory.
One of the topics you most often write about is the concept of narrative. In today’s market, it often isn’t the actual story that matters, but rather the story that people tell about the story. For example, the Federal Reserve did pretty much what everyone expected it to do in 2018, but the changes in the narrative surrounding what they did had a major impact on the market in both directions. I was wondering if you could talk about why narratives have become so important in the market and what you think it means for the future of investing.
Ben: Sure. Happy to. It’s a new way of describing an old
idea and what I’m describing is very similar to what, of all people, John
Maynard Keynes described investing was like in the 1930s, which I think was
probably the last time when fundamentals became so divorced from the market
prices that we all endure. And when you read Keynes’ magnum opus, The General Theory of Employment, Interest and Money,
where he lays out all of his big macroeconomic ideas, he’s also got a couple of
chapters there on what he’s doing with his actual investments, because this was
written during a period of time when there wasn’t this enormous gulf between the
academic aspect of economics and macroeconomics and the very practical aspect
of managing money.
So Keynes ran the endowment for King’s College at Oxford, and actually did
really well with that. And he’s got a couple of chapters on what it’s like to
actually try to make money in this environment. And when he talks about that,
and this gets picked up in some of his later writings and other people have
noted this, he talks about what he calls the newspaper beauty contest. And what
he’s talking about there was very popular in the day. We didn’t have American Idol
or these popular social media at the time. You had newspapers. And so its kind
of similar to American idol. The newspapers would run these contests where they
would publish the pictures of 12 pretty girls, and they’d invite their readers
to vote on who they think is the prettiest girl. So if you voted for the pretty
girl who got the most votes, then you would be entered in a drawing. Whoever
got picked out of that hat would get an all-expense paid trip to Atlantic City
or something like that. And the way you played that game when you thought about
it, you’re not going to vote for who you actually think is the prettiest girl.
So that would be what Keynes would have described as first-degree decision
making. What I’m really trying to do is I’m trying to vote for, I want to get
into this pool to win the sweepstakes.
So it’s not really who I think is the prettiest girl. It’s
who everyone else thinks is the prettiest girl. So now we’re talking about
second degree decision making. I want to vote for who the consensus would be as
the prettiest girl. And so you’re thinking about that and you think, well, you
know, who do I think is going to be the consensus and then all of a sudden it
hits you. Wait a second, am I the only one who is smart enough to figure out
how this game is played? Or I’ll put it another way, is everyone so dumb that
they’re going to be voting for who they really think is the prettiest girl? Of
course not, right? We’re all smart enough to figure out how the game is played
given these rules. And so it’s not who we think is the prettiest girl. It’s not
who we think is the consensus prettiest girl.
But we realize that all of us are thinking that way. It’s all of us thinking
about who do all of us think is the prettiest girl. And that’s what Keynes
called this third level of decision making. He said that’s what markets are
like today. That when fundamentals don’t seem to matter as much, when it’s
external policy that seems to really drive markets, then we start thinking not what
stock do we think is the prettiest stock. Or the prettiest company. Or not even
what’s the consensus. We start thinking about what’s the consensus of the
consensus. And he said, that’s the game we have to try to figure out.
And modern game theory calls this the common knowledge game and the way you can try to get ahead of the common knowledge game and try to figure out the consensus of the consensus is actually to look at what we call our missionaries in game theory. Missionaries are the people who say this should be your choice as the prettiest girl, right? It’s not because you agree with them or not. Its because it provides a focal point for all of us trying to understand the consensus of the consensus. Whether we think that’s the prettiest girl or not, it doesn’t matter. We know this is how the game is played. So that’s why this focus on the narrative. I think it’s so important because what we’re really focusing on is the effort that opinion leaders or missionaries as we’re calling it, we’re looking at the effort that they make to try to focus public opinion on an answer for what is the, not the prettiest girl, but the prettiest stock or the prettiest sector or the prettiest strategy. And that’s at the core of what we’re trying to do.
Jack:
Although narratives may seem to many like something that can’t be quantified,
the rise of Artificial Intelligence and Natural Language Processing actually
opens up opportunities to develop models to try to quantify narratives and use
them to make investment decisions. In your excellent article on this topic on
Epsilon Theory, you talked about the concepts of attention, cohesion, and
sentiment and how they can be used to potentially develop an investment
strategy based on narrative. Can you talk about how that might work?
Ben: I’d be happy to. So those three concepts are, we think are just as you described. There’s attention. There’s call it cohesion. And then there’s
So it’s not as straight forward as you might think. Even
just to measure whether a word is a positive or negative word, but even if you
do get a rough sense of the positivity or the negativity, that’s the least
important aspect of how powerful the impact on an investment crowd a narrative
will be. And I say that because there’s nothing about sentiment that captures,
let’s call it the strength of the drum beat of a narrative. And that’s
attention. There’s nothing about sentiment that captures the focus of a
narrative. And that’s cohesion. What we’re really trying to measure here much
more than sentiment is the structure of a narrative. Because its when you look
at the structure that you can really see a narrative being born, grow, maybe
evolve or split into different narratives, and frankly ultimately die out.
You really have to look at and visualize these other structural attributes like
attention, which is like the volume of the narrative. And then cohesion, which
is the focus of the chords that are being played by the narrative. You have to
put all three of these together, we think, before you can really understand
this process of focusing the investment crowd and their behavior on the
consensus of the consensus or the story behind the story for a stock or a
security or a sector.
Jack: Its interesting because it seems like what you are doing relates to factor investing in one way in that you are not trying to use your opinion about the narrative, but are instead trying to do this based on math.
Ben: That’s exactly right. That’s exactly right. It’s the principles that we’re, that we’re describing here, these notions of, of attention and cohesion, even sentiment. These are not new ideas. The basic, I’ll call it game theory behind LDS was put forth by John Maynard Keynes back in the 30s. The math here that we’re describing was all I’ll call it, discovered. It was all written down and published widely in linguistics and economics and even anthropology. All the math here was published 20 or almost 30 years ago. What’s been missing that’s available today is just the raw shear computer processing power that you really have to have to make this work.
So these calculations that we make in comparing, you know, one Bloomberg article to another Bloomberg article, the calculations themselves are not complicated. You’re just basically, you’re comparing words, right? Or with what we call in
So, you know, it’s just comparing one article with a thousand
Jack: It has been said that “this time is different” is the most dangerous phrase in investing, but in reality, “this time is never different” can be equally as dangerous.
In your article The Three Body Problem, you talk about how the introduction of a third body to a system of two bodies moving in space makes the future movement of all three of them completely unpredictable. Your thesis in the piece is that the Federal Reserve’s purchase of massive amounts of financial assets has become that third body in the world of
Ben: Sure. And, and it all gets back again to the basic idea that I was describing earlier that none of these are really new ideas. These are old ideas, but we have a new technology to try to understand them. And in exactly the same way that an investor in the 1930s, like John Maynard Keynes was talking about how the ground wasn’t steady beneath their feet, fundamental investors, particularly value investors, were just getting slaughtered in the 1930s. You know, in the same way that he was describing that you had a very policy driven investment world, we have the same situation going on today. So it’s not that this time is different. My point is that things are always different, right?
And what we think is some permanent feature of the investment world, like fundamentals matter, that’s what’s not true. That certainty is a really false certainty. And I call it the three body problem because it’s a very famous physics problem that was solved by Henri Poincaréin the early 20th century. The three body problem says that if you’ve got three, let’s call them planets that are circling around in space, and those are the only three bodies in the universe. And they’ve all got gravity of course. So they’re rotating or they’re spinning around each other. With the exception of some very peculiar situations, what Poincaré proved was that there is no algorithm that can predict the position of those three bodies at some point in the future. You can’t. There’s no formula. There’s no formula where you can plug in their current position, their mass, their speed, and you can just punch the algorithm to predict where there’ll be two minutes from now or two years from now. Now you can estimate it, right? You can calculate it. You can say, okay, I know where they were a second ago. I know how these bodies act on each other. And so I can estimate where they’re going to be a second from now and then a minute from there, you know, do all that. But there’s no formula. There’s no algorithm that can tell us where they’re going to be in the future. And you know, as the kids would say, it’s just math, right? It’s a provable thing.
And the reason I think that’s so important for when we think about investing is that we don’t have just three bodies, but we’ve got a lot of big important centers of gravity, right? You know, bodies that exert gravity on us as investors. One of them is the fundamentals of a company, the fundamentals of an economy, right? It exerts a lot of gravity. But sometimes, as happened in the aftermath of the great financial crisis, we get a whole new center of gravity that comes in. In this case, central banks buying $20 trillion (trillion with a t) dollars worth of stuff, of financial assets. And $20 trillion creates an enormous amount of gravity. And so what I’m saying is that in exactly the way that Poincaré proved, there is no algorithm, there is no model that can predict the future when you’ve got these big centers of gravity that are spinning around each other. Well that’s what happened to us. We have this enormous new center of gravity that came up. And so what I am saying is that fundamentally, mathematically, whatever model we think we had for how stocks work, it’s not going to work. It’s just not going to work. What we have to do is take a very different approach. We have to think about how do we calculate it, not how do we predict it. And it’s a tough kind of concept I find to, to wrap one’s head around because we’re always in the business of trying to find the model. We’re trying to find the secret formula.
Well, the formula that worked for the last ten years, it may not work for the next ten years. It will not work for the next 10 years if you’ve got a new center of gravity here that’s exerting its force on everyone. So I get excited and long winded about this stuff, but it’s an old idea that when we think about it with kind of our new tool kits, the computer processing power we have; it’s not a depressing idea to me to think that we have to calculate rather than predict. It’s actually pretty exciting to me because I do think that we have the tools where we can do some of these calculations and that leads us to a much more agnostic and yet more powerful way of understanding the future of any complex system including investing.
Jack: When you wrote that article, it really made me think about the concept of base rates. Value investing has been underperforming for a really long time, and every value investor (myself included) tends to look to the past for the implications of that. And the past shows that every time you have had a decade long period of underperformance like this, you had strong returns in the next decade. But your article made me think about how much weight I can put in that past data given that we now have this new body floating around making the future unpredictable. I was wondering if you could talk about what the outlook for factor investing is in this new world.
Ben: Sure. So my big point here is that these factors exist. I’m not saying that value doesn’t work. What I’m saying is that when you get a new, when you get a structural change in
Jack: So it’s not that factors necessarily won’t work. It’s that it’s going to be to some degree, random and we don’t know which ones are going to work. We don’t know when they are going to work?
Ben: Absolutely correct. We don’t know when they’re going to work. So. Exactly right. It’s observable. It’s observable. And, and I do think that if you apply a lot of these calculation tools and calculation engines, which is big data and the power of big compute, I think you can get some insights into how it is evolving in the very near term. But it’s not like there’s some magic formula, like a mean reversion formula that in some certain period of time it is reverting back to some prior period of time. That is what does not work.
Jack: If you were going to be a factor investor in this type of world what it seems you might want to do is apply all of them rather than bet on any given factor mean reverting. Doing that might give you the best chance of success going forward.
Ben: Correct. I think it does.
Jack: Your recent Twitter post that argued that alpha is not possible without non-public information created a lot of controversy, which I guess is to be expected since active managers need to defend their ability to produce alpha to justify their existence. Can you explain what you mean when you say that there is no alpha without non-public information?
Ben: Well without private information, which is a little different. Let me say this, though. So a lot of people I think took this to mean that Alpha could only come from material nonpublic information, MNPI, so insider information. That’s not it. The relationship between MNPI and private information is the relationship between squares and rectangles. All squares are rectangles, All MNPI is private information, but not all rectangles are squares. Not all private information is MNPI. In other words, there is private information, there is alpha beyond MNPI, you know, illegal information. Its just incredibly rare.
What I was really reacting to is this idea of that, and frankly I’ll say I see this a lot with value investors, right? Where they say “oh, if I can just read those Q’s and K’s and I think really hard about the company, then that gives me an edge, right. And that that’s what I’m reacting to. There is no edge in thinking really hard about public information. There’s no edge there.
Jack: If you look at this from the perspective of the different types of edges in investing, it seems as if you are saying that the only way to produce alpha is via an informational edge. Does that mean that it is not possible to better analyze the information that is publicly available than other market participants to produce an analytical edge?
Ben: An analytical edge on public information is not
What they were doing was basically options arbitrage using
the Black Scholes formula, which had not been made public, but they had
discovered privately. So they were looking at public information; options data
and the like, but they had discovered this formula, the Black Scholes formula,
for squeezing information out of that public data that was entirely private.
That was entirely their own. So when I say there’s no analytical edge possible,
what I’m saying is that there’s no analytical edge possible from humans
thinking about stuff.
You have to actually invent a new tool to do some analysis like a Black Scholes
formula.
Jack: So if you think of a firm like RenTech, that would probably explain a lot of what they are doing, right?
Ben: Correct. And frankly it’s what I’m trying to do as well. So when I’m talking about this natural language processing and the tools that we’re developing here, you know, these are all tools that we’re applying to public information, right? To newspaper articles and Wall Street publications and the like. But I do think that there is private information that can be found both from, as you’re calling it an informational advantage – I’m going to go and count all the cars and the parking lot at Walmart – that’s information that has not been publicly distributed. And so that’s legal private information. I also think you can invent things like a Black Scholes formula or I think some of these, the stuff we’re working on, they can give you private information as well from public sources.
Jack: One of the biggest problems that trips up many investors is an unwillingness to change their views, even in the face of changing facts. In the podcast you did with Patrick O’Shaughnessy, you talked about how often the most difficult conversation an investor can have is the one with themselves. You have been able to evolve your views over time as you have seen things change in the market and your work at Epsilon Theory shows a willingness to challenge conventional wisdom in many areas. Do you have any advice you can give average investors for how they can develop a mindset that allows them to overcome their confirmation bias and develop the flexibility to see the world around them for what it is, and not what they think it should be?
Ben: So there’s this famous book and the idea that people are either foxes are hedgehogs, right? And the difference is that the hedgehog has one big idea and the foxes have lots of small ideas. And I’ve always been a fox, not a hedgehog. And so I find it comes more easily to me then I think a lot of people, or some people. I think there are a lot of people like me; foxes as opposed to hedgehogs. So I really do think that there is some kind of difference between people and their willingness or their ability to adjust or to adopt what I was describing earlier as a philosophy of profound agnosticism. To really confront the notion that you really don’t know and we can’t know certain things. That’s so hard in this business, right? Because it’s so hard to make a living with profound agnosticism because, particularly in the investment world, there’s always someone out there who, if you tell a client, I don’t know, your client will find plenty people who tell them I do know, right? Come work with me because I do know, I do know the answer. We all want to know the answers. And so that’s the hardest thing in the world from a business perspective to say, “I don’t know”. But I think to have that sort of authentic relationship with a client or with a boss or whoever you have to report to, it’s imperative to develop and practice those simple words. I. Don’t. Know.
Jack: Thank you again for taking the time to talk to us today. If investors want to find out more about you and Epsilon Theory, where are the best places to go?
Ben: Our website is epsilontheory.com. You can also find me on Twitter at @epsilontheory and my partner Rusty Guinn at @wrguinn.
Photo: Copyright: 123rf.com/guliverislt